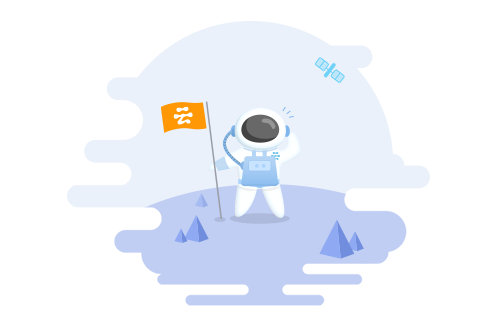
{"product":"PRODUCTS","solution":"SOLUTIONS","keyword":"KEYWORDS"}
0.0.201
A comprehensive suite of global cloud computing services to power your business
Payment Methods We Support
Live-chat with our sales team or get in touch with a business development professional in your region.