Resource demand is difficult to predict in cloud native scenarios. Horizontal Pod Autoscaler (HPA) provided by Kubernetes scales resources with a scaling delay and the configuration is complex. To resolve the preceding issues, ACK released Advanced Horizontal Pod Autoscaler (AHPA), which is powered by time series intelligence from DAMO Academy. AHPA can automatically learn the pattern of workload fluctuations and predict resource demand based on historical metric data to help you implement predictive scaling. This topic describes the business architecture, advantages, and scenarios of AHPA.
Background information
The following traditional methods are used to manage the pods of an application: manually specify the number of pods, use HPA, and use CronHPA. The following table describes the disadvantages of the preceding methods.
Method | Disadvantage |
---|---|
Manually specify the number of pods | Resources are wasted during off-peak hours. Idle resources are still billed. |
HPA | Scaling activities are performed after a scaling delay. Scale-out activities are triggered only if the resource usage exceeds the threshold and scale-in activities are triggered only if the resource usage drops below the threshold. |
CronHPA |
|
- Traditional horizontal pod scaling: Scale-out activities are triggered after the amount of workloads increases. The system cannot provision pods at the earliest opportunity to handle the fluctuating workloads due to the scaling delay.
- Predictive horizontal pod scaling: AHPA learns the pattern of workload fluctuations based on the historical values of specific metrics and the amount of time that a pod spent before the pod entered the Ready state. This way, AHPA can provision pods that are ready to be scheduled before a traffic spike occurs. This ensures that resources are allocated at the earliest opportunity.
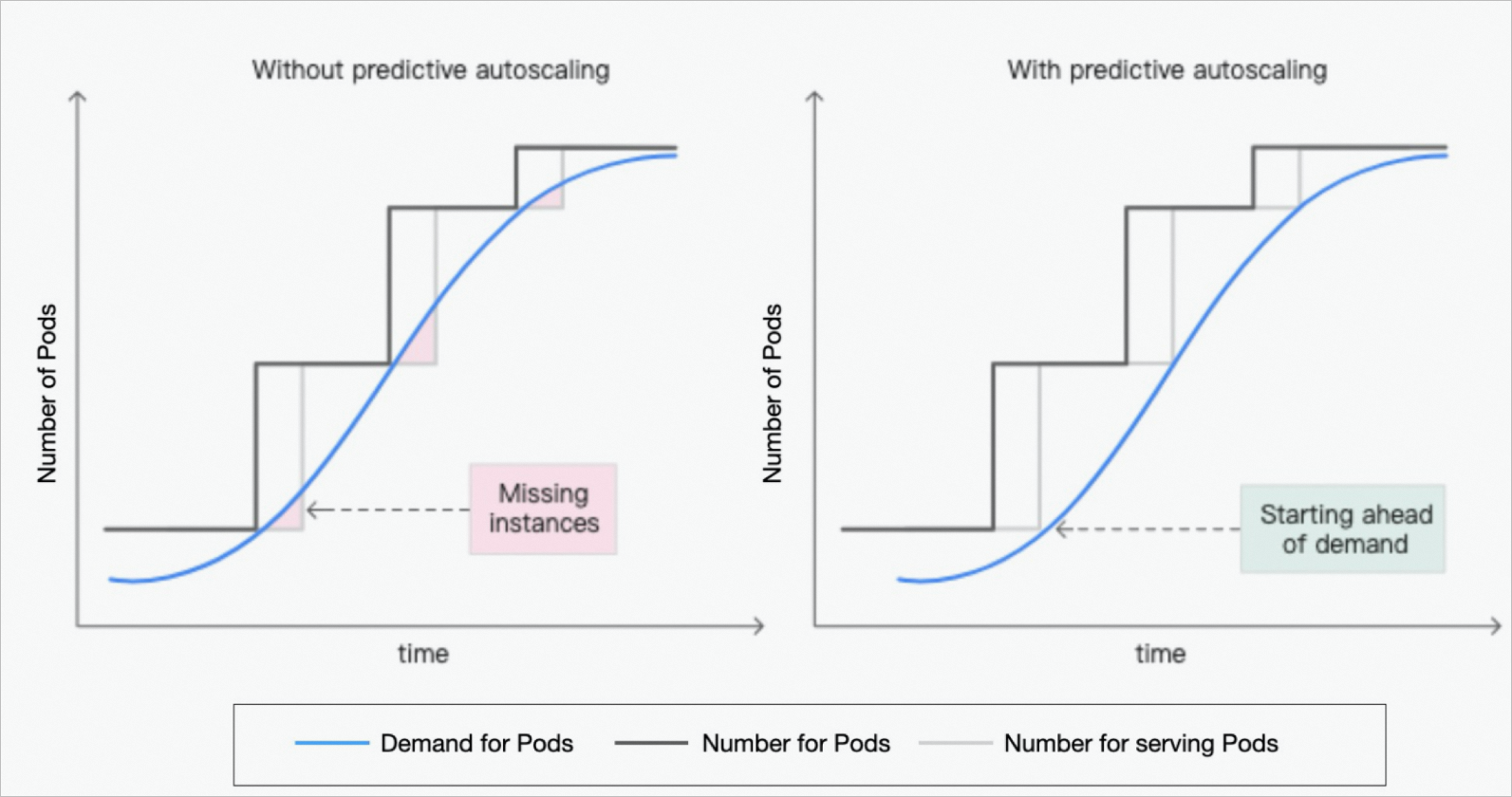
Business architecture
- Various metrics: supports metrics such as CPU, GPU, Memory, QPS, RT, and external metrics.
- Stability: uses proactive prediction, passive prediction, and service degradation
to guarantee sufficient resources for applications.
- Proactive prediction: predicts the trend of workload fluctuations based on historical metric data. Proactive prediction is suitable for applications whose workloads periodically fluctuate.
- Passive prediction: predicts workload fluctuations in real time. AHPA can predict workload fluctuations and deploy resources in real time.
- Service degradation: allows you to specify the maximum and minimum numbers of pods within one or more time periods.
- Multiple scaling methods: AHPA can use Knative, HPA, and Deployments to perform scaling.
- Knative: AHPA can help resolve the cold start issue in resource scaling based on concurrency, QPS, or RT in serverless scenarios.
- HPA: AHPA can simplify the configuration of HPA scaling policies and help beginners handle the scaling delay issue.
- Deployment: AHPA can directly use Deployments to perform auto scaling.
Advantages
- High performance: AHPA can predict workload fluctuations within milliseconds and scale resources within seconds.
- High accuracy: AHPA can learn complex patterns of workload fluctuations with an accuracy higher than 95% based on proactive prediction and passive prediction.
- High stability: AHPA allows you to specify the maximum and minimum numbers of pods required within time periods that are accurate to minutes.
Scenarios
- Applications whose workloads periodically fluctuate, such as live streaming, online education, and gaming applications.
- Scenarios in which a fixed number of pods are deployed and auto scaling is also used to handle workload fluctuations.
- System recommendations on the number of pods to be provisioned are required. AHPA provides a Kubernetes API to allow you to obtain prediction results. You can integrate the API into your business systems.
References
- For more information about how to deploy and use AHPA, see Deploy AHPA.
- For more information about how to use AHPA to perform predictive scaling based on GPU metrics, see Use AHPA to perform predictive scaling based on GPU metrics.
- For more information about how to use AHPA to enable predictive scaling in Knative, see Use AHPA to enable predictive scaling in Knative.