This topic describes the MapReduce API supported by MaxCompute and its limits.
MaxCompute provides two versions of the MapReduce API:
- MaxCompute MapReduce: the native MapReduce API. This version runs fast. It is convenient to develop a program without the need to expose file systems.
- Extended MaxCompute MapReduce (MR2): an extension of MaxCompute MapReduce. This version supports complex job scheduling logic. The implementation method is the same as that of MaxCompute MapReduce.
Note You cannot use MapReduce to read data from or write data to external tables.
Scenarios
MapReduce supports the following scenarios:
- Search: web crawl, inverted index, PageRank.
- Analysis of web access logs:
- Analyze and summarize the characteristics of user behavior, such as web browsing and online shopping. The analysis can be used to deliver personalized recommendations.
- Analyze user access behavior.
- Statistical analysis of texts:
- Word count and term frequency-inverse document frequency (TFIDF) analysis of popular novels.
- Statistical analysis of references to academic papers and patent documents.
- Wikipedia data analysis.
- Mining of large amounts of data: mining of unstructured data, spatio-temporal data, and image data.
- Machine learning: supervised learning, unsupervised learning, and classification algorithms, such as decision trees and support vector machines (SVMs).
- Natural language processing (NLP):
- Training and forecast based on big data.
- Construction of a co-occurrence matrix, mining of frequent itemset data, and duplicate document detection based on existing libraries.
- Advertisement recommendations: forecast of the click-through rate (CTR) and conversion rate (CVR).
Process
A MapReduce program processes data in two stages: the map and reduce stages. It executes the map stage before the reduce stage. You can specify the processing logic of the map and reduce stages. However, the logic must comply with the conventions of the MapReduce framework. The following procedure shows how the MapReduce framework processes data:
- The MapReduce framework partitions data and uses data in each partition as the input for a mapper. After data is partitioned, multiple mappers start to process the data at the same time.
Before the map operation, the input data must be partitioned. Partitioning refers to the splitting of the input data into data blocks of the same size. Each data block is processed as the input for a single mapper. This allows you to use multiple mappers at the same time.
- Each mapper reads its partition data, computes the data, and generates data records to a reducer. When a mapper generates data records, it must specify a key for each data record. A key specifies the reducer to which a data record is sent. Keys and reducers share a many-to-one relationship. Data records with the same key are sent to the same reducer. A reducer may receive data records with different keys.
- Before the reduce stage, the MapReduce framework sorts data records based on keys to ensure that data records with the same key are adjacent. If you specify a combiner, the MapReduce framework calls the combiner to combine data records that share the same key. You can define the logic of the combiner. Different from the classic MapReduce framework protocol, MaxCompute requires that the input and output parameters of a combiner must be consistent with those of a reducer. This process is called shuffle.
- At the reduce stage, data records with the same key are transferred to the same reducer. A single reducer may receive data records from multiple mappers. Each reducer performs the reduce operation on multiple data records with the same key. After the reduce operation, all data records with the same key are converted into a single value.
- The results are generated.
Note For more information about the MapReduce framework, see Features.
The following section uses WordCount as an example to explain the related concepts of MaxCompute MapReduce at different stages.
Assume that a file named a.txt exists and each line of the file contains a digit. You want to count the number of times each digit appears. Each digit is called a word, and the number of times it is used represents the count. The following figure shows how MaxCompute MapReduce counts the words.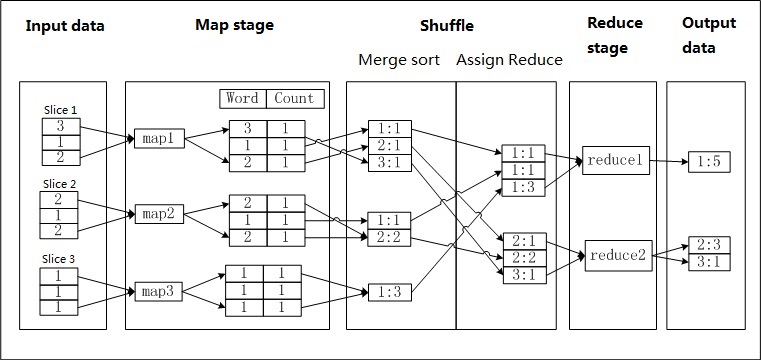
Procedure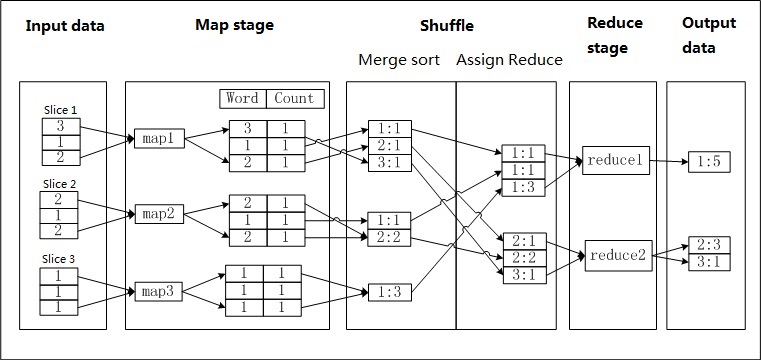
- MaxCompute MapReduce partitions data in the a.txt file and uses data in each partition as the input for a mapper.
- The mapper processes input data and records the value of the Count parameter as 1 for each obtained digit. This way, a <Word, Count> pair is generated. The value of the Word parameter is used as the key for the newly generated pair.
- At the early shuffle stage, the data records generated by each mapper are sorted based on keys (the value of the Word parameter). After the data records are sorted, the records are combined. This requires that you accumulate the Count values that share the same key to generate a new <Word, Count> pair. This is a merging and sorting process.
- At the late shuffle stage, data records are transferred to reducers. The reducers sort the received data records based on the keys.
- Each reducer uses the same logic as the combiner to process data. Each reducer accumulates the Count values with the same key (the value of the Word parameter).
- The results are generated.
Note All the MaxCompute data is stored in tables. Therefore, the input and output of MaxCompute MapReduce can be only in the table format. You cannot specify the output format, and no interfaces similar to file systems are provided.
Limits
- For more information about limits on MaxCompute MapReduce, see Limits on MaxCompute MapReduce.
- For more information about the limits on the running of MaxCompute MapReduce in local mode, see Local run.